Overcoming Data Labeling Challenges is vital for AI Project success, as high-quality labeled data drives effective machine learning models. Combining manual and automated labeling methods, like active learning, improves efficiency and accuracy. Collaboration between human annotators and AI algorithms ensures better handling of complex and unstructured data. By Streamlining Labeling Tasks and focusing on data quality, organizations can build robust AI applications and enhance model performance, laying a strong foundation for future success.
What is Overcoming Data Labeling Challenges and Why is It Important for AI?
AI models receive accurate, high-quality training data, which is crucial for reliable performance. Managing these challenges with manual and automated approaches reduces errors, enhances efficiency, and prevents bias. Successful data labeling directly impacts AI’s ability to learn, predict, and make informed decisions, driving better outcomes across applications like NLP and image recognition.
Benefits of Automating Data Labeling Processes
Benefit | Description |
---|---|
Enhanced Accuracy | Comprehensive data improves the precision of automated systems. |
Optimized Efficiency | Reduces manual workload, speeding up the labeling process. |
Adaptability | Regular updates ensure relevance with changing environments. |
Scalable Solutions | Handles large volumes of data seamlessly. |
Cost-Effective | Reduces operational costs by minimizing human intervention. |
Consistency | Ensures uniform labeling across diverse datasets. |
What Are the Best Practices for Effective Data Labeling?
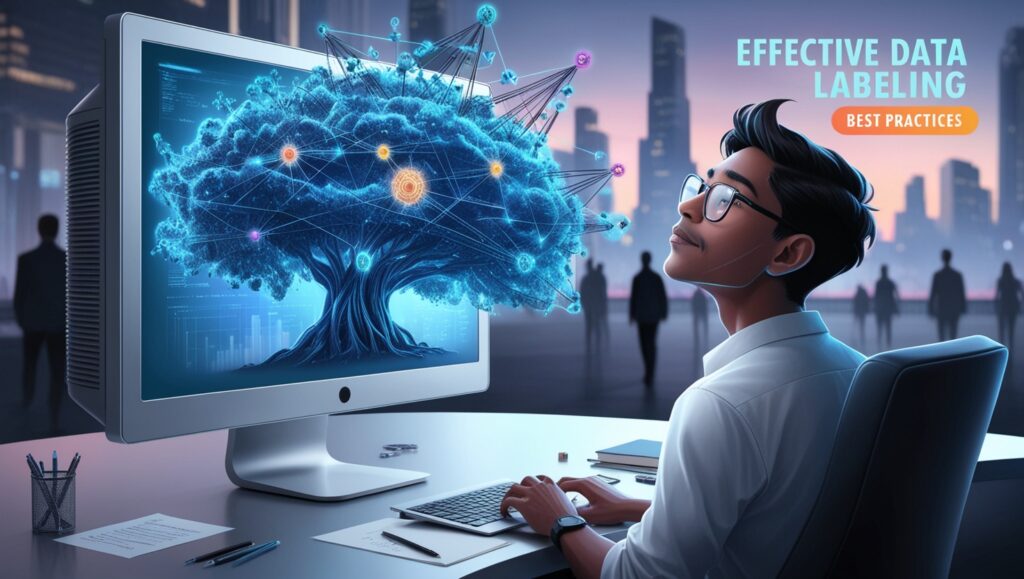
- Establish clear guidelines to maintain label consistency.
- Combine automated tools with human oversight for accuracy.
- Invest in labeler training to improve understanding and efficiency.
Workflow for Efficient Data Annotation
- Define clear guidelines for consistent annotations.
- Use collaboration tools to enhance team communication.
- Conduct regular quality checks to ensure high accuracy.
Quality Control Measures for Labeled Datasets
- Implement validation procedures to identify errors and inconsistencies.
- Perform regular audits and peer reviews to improve dataset integrity.
- Continuously refine processes to maintain high standards.
Training Labelers for High-Quality Annotation
- Provide clear guidelines and practical examples.
- Offer regular feedback and skill assessments.
- Focus on consistency to enhance annotation precision.
What Future Trends Are Shaping Data Labeling in AI?
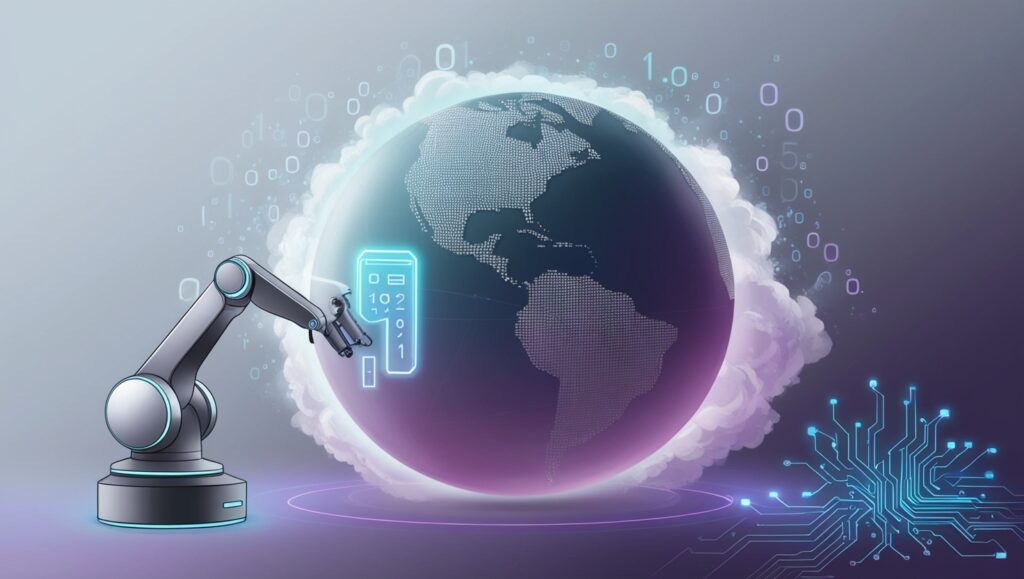
Automation, crowdsourcing, and advanced machine learning algorithms are driving data labeling trends, improving efficiency, enhancing label accuracy, and enabling faster, semi-automated processes.
Impact of Machine Learning on Data Labeling Strategies
Strategy | Description | Benefits |
---|---|---|
Manual Labeling | Human annotators label data points manually. | High precision but time-consuming. |
Automated Labeling | ML algorithms automate labeling processes. | Increases speed and efficiency. |
Semi-Supervised Learning | Uses a small set of labeled data to guide the labeling of larger datasets. | Reduces labeling effort and costs. |
Active Learning | Prioritizes labeling the most informative data points. | Optimizes labeling for better model performance. |
In Conclusion
Overcoming Data Labeling Challenges is essential for the success of AI projects. By combining manual efforts with automated processes, organizations can enhance accuracy, efficiency, and scalability in data labeling. Emphasizing best practices, continuous training, and robust quality control will ensure high-quality labeled datasets. As automation and advanced techniques evolve, the ability to effectively navigate these challenges will lay a strong foundation for reliable AI applications, ultimately driving better outcomes across various domains. Prioritizing this aspect will empower teams to build innovative solutions and maximize the potential of machine learning.