With WebXMeta’s cutting-edge Data Labeling Tools, you’ll be at the forefront of efficient and precise data labeling. In today’s data-driven world, high-quality labeled data is critical for training machine learning models across a wide range of applications. WebXMeta meets this demand by providing a collection of powerful tools meant to automate the data labeling process while maintaining precision and consistency in every annotation. Our platform provides users with simple tools for picture and text annotation, object detection, semantic segmentation, and more. WebXmeta optimizes annotation workflows by leveraging cutting-edge AI technology, allowing professionals and novices to cooperate effortlessly in generating labeled datasets of unprecedented quality. Join us in shaping the future of AI training data with webXmeta’s Data Labeling Tools, where innovation meets precision.
What are Data Labeling Tools?
Data Labeling Tools are important in the field of machine learning because they help with data labeling for training and improving machine learning models. A data labeling tool, also known as a label tool, is used to label data by labeling each data point by tagging or categorizing it. This labeled data is then used to train machine learning models, which use the labeled examples to predict unknown data. Data labeling tools handle image categorization, object detection, sentiment analysis, and other labeling tasks. They include a number of tools and capabilities for tagging various sorts of material, including text, photos, audio, and video. Furthermore, these technologies provide a variety of data labeling options, including manual, semi-automated, and automatic labeling, providing flexibility and scalability in the labeling process. Overall, data labeling technologies improve machine learning models accuracy and performance by providing high-quality labeled data for training.
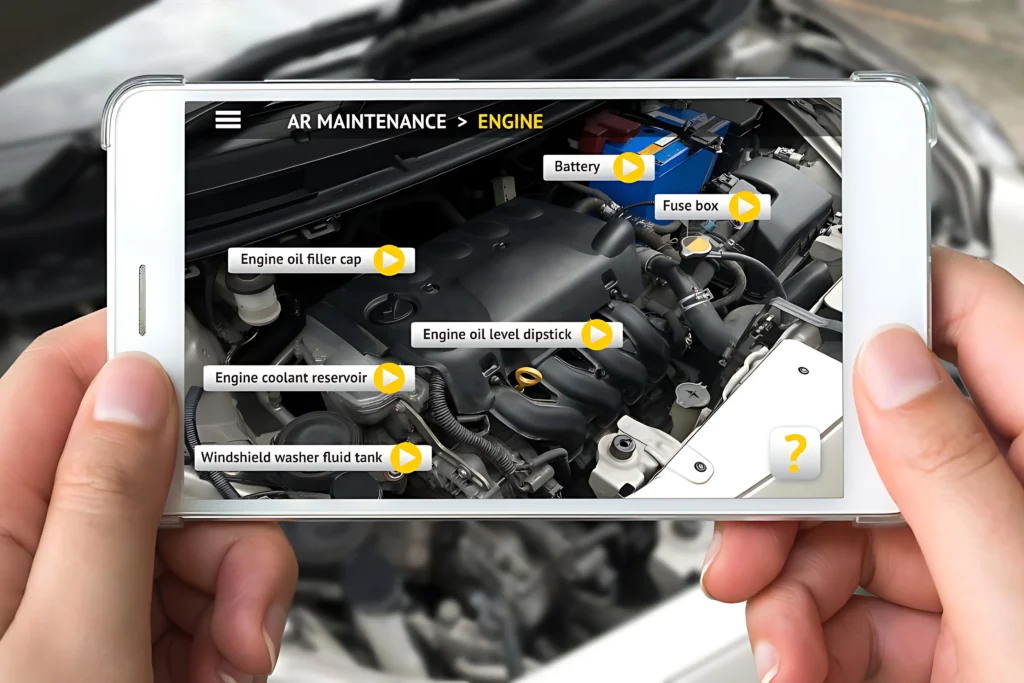
How to Choose the Right Data Labeling Tool?
Choosing the right data labeling tool is crucial for organizations embarking on machine learning projects. A data labeling tool is a software application that helps annotate and label data for training machine learning models. The process of data labeling is a critical step in machine learning as it provides labeled data that acts as a reference for the model’s learning. When considering a data labeling tool, one should evaluate its functionality, ease of use, and scalability. An ideal label tool should accept numerous data formats and provide a number of annotation choices. It should also provide features for collaboration, quality control, and data management. Additionally, organizations should assess if the tool allows for automatic data labeling, which reduces the manual effort required in the labeling process. Overall, selecting the right data labeling tool is essential for ensuring accurate and efficient machine learning model training.
Top Data Labeling Tools
In the realm of machine learning, data labeling plays a crucial role in training models to make accurate predictions. To facilitate this process, there are various top data labeling tools available in the market.
- Label Studio is an open-source annotation tool that allows users to label data for training machine learning models. With an intuitive interface and support for various annotation types, Label Studio proves to be a versatile data labeling tool.
- Labelbox, which offers a comprehensive platform for creating and managing training data. Its features include automatic data labeling, collaboration tools, and integrations with popular machine-learning frameworks.
- SuperAnnotate is yet another efficient data labeling tool, providing a user-friendly interface and seamless integration with machine learning workflows.
- VGG Image Annotator (VIA) and RectLabel are also prominent contenders in the data labeling landscape, offering robust annotation capabilities for different labeling scenarios.
These top five data labeling tools provide invaluable support for users seeking to label data and enhance the performance of their machine-learning models.
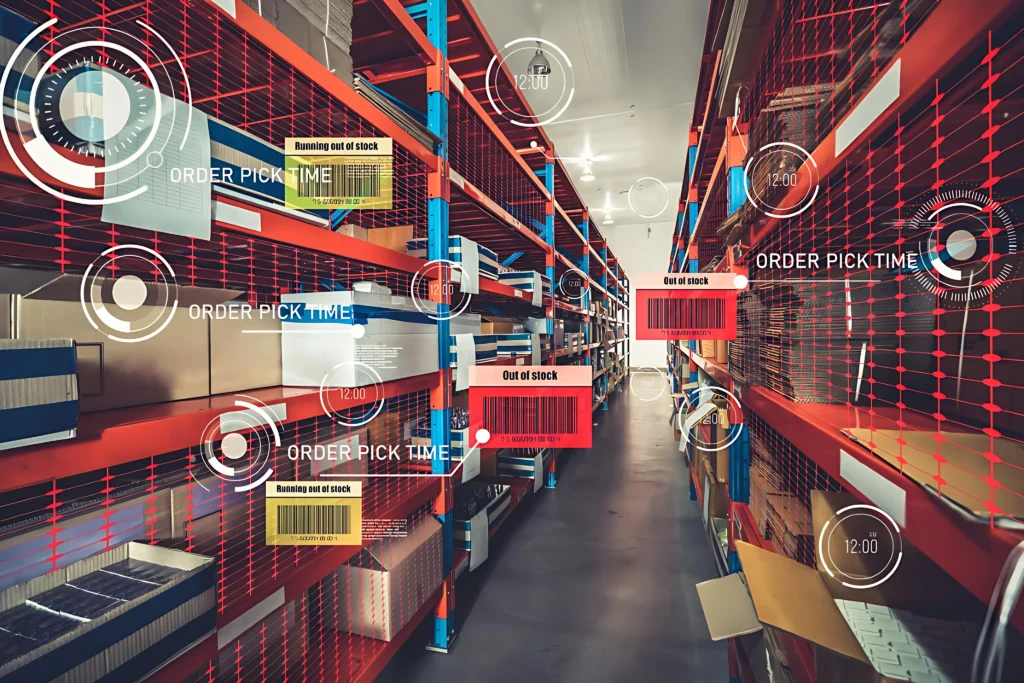
The major difference between Data Labeling and Data Annotation
Data labeling and data annotation are two essential processes in developing machine learning models. While they may seem similar, there are significant differences between the two.
Sr. No | Data Labeling | Data Annotation |
---|---|---|
1 | Data labeling refers to the process of assigning descriptive tags or labels to specific data points using a label tool. Conversely. | Data annotation involves the task of adding meaningful annotations, such as bounding boxes or key point labels, to visual or textual data using an annotation tool. |
2 | Data labeling focuses on labeling the training data required for training a machine learning model. | Data annotation extends beyond training data to encompass broader data tasks like object detection or sentiment analysis. |
3 | Data labeling often involves human annotators who interpret the data and apply labels based on guidelines. | Data annotation can sometimes involve automatic data labeling techniques, which use algorithms to label the data automatically. |
4 | The primary goal of data labeling is to recreate the ground truth. | Data annotation aims to provide contextual information for better understanding and analysis. |
5 | Data annotation is necessary for evaluating and improving the model’s performance. | data annotation is necessary for evaluating and improving the model’s performance. |
6 | Top data labeling tools, such as Label Studio, are widely used for data labeling tasks. | Data annotation tools like RectLabel or VGG Image Annotator (VIA) are popular for data annotation tasks. |
Conclusion
Data Labeling and Data Annotation are distinct processes that play integral roles in the development and refinement of machine learning models.
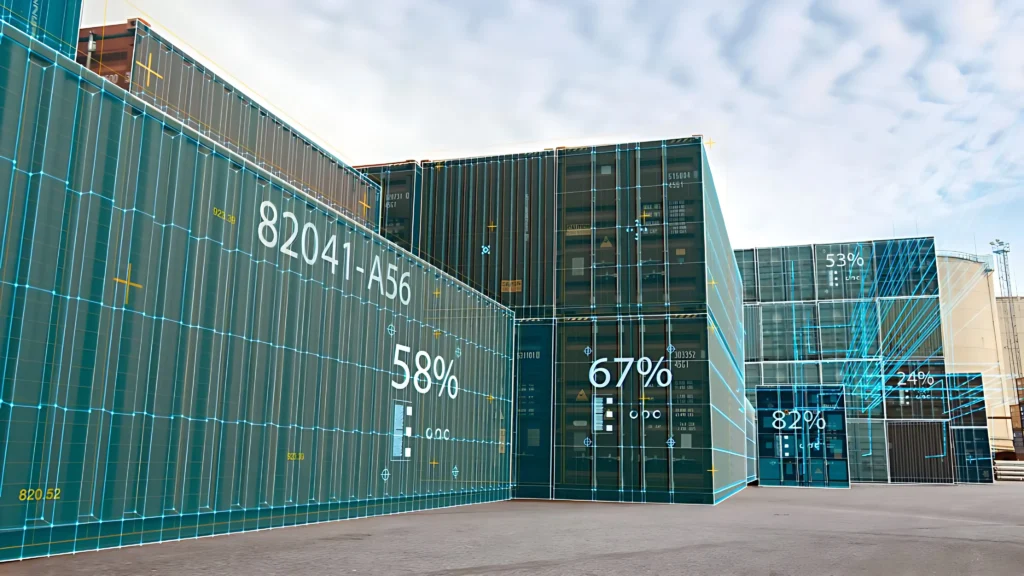
If you’re looking for a Data Labeling Tool to help you level up your process
There are several solutions to consider if you need a data labeling tool to improve your operation. A data labeling tool is a useful tool for accurately and efficiently identifying various forms of data, such as text, photos, or video. This tool is essential for producing high-quality training data, which is a prerequisite for successful machine-learning models. You may streamline your data labeling process by employing a dependable label tool, reducing time and maintaining consistency in the annotations. Additionally, good Data Labeling Tools allow for collaboration among team members, facilitate the allocation of tasks, and ensure a smooth workflow. When dealing with video data, a Labeling tool that can handle video annotation tasks such as object tracking or activity recognition becomes particularly beneficial. Therefore, investing in a Data Labeling tool is an essential step towards improving the accuracy and efficiency of your machine learning projects.
Access a Data Labeling Workforce at WebXMeta
Webxmeta provides a reliable and efficient solution for accessing a highly skilled and experienced data labeling workforce. With its extensive network of trained annotators, Webxmeta ensures accurate and high-quality labeling for various types of data, including images, text, and audio. Their platform offers a user-friendly interface that allows users to easily manage and track the progress of labeling projects. Webxmeta’s team is committed to providing outstanding customer service and attempts to fulfill the unique needs of each client. By partnering with Webxmeta, businesses can access a flexible and scalable labeling workforce, saving time and resources. Whether it’s for training machine learning models, improving search algorithms, or data analysis, Webxmeta delivers precise and reliable data labeling services that enhance the efficiency and performance of AI systems.